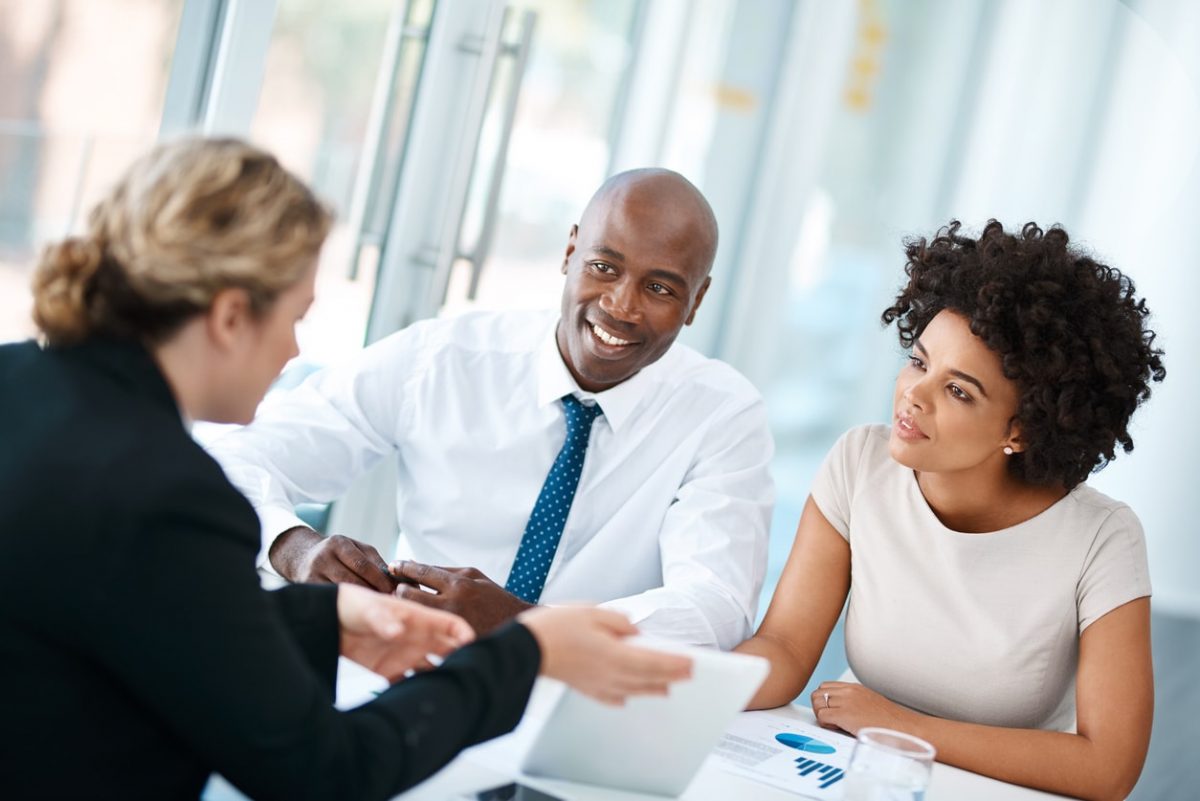
Обо мне
How to Calculate Sensitivity: A Clear and Confident GuideCalculating sensitivity is an important aspect of medical diagnosis. Sensitivity is a statistical measure used to determine the accuracy of a diagnostic test. It is the proportion of true positive results among all those who actually have the condition being tested for. In other words, sensitivity measures how well a test can correctly identify individuals who have the condition.
Sensitivity is an important measure for diagnostic tests because it helps to determine the reliability of the test. A test with high sensitivity is able to correctly identify most individuals who have the condition being tested for. On the other hand, a test with low sensitivity may miss a significant number of individuals who actually have the condition. Therefore, it is important to calculate sensitivity accurately in order to ensure the appropriate diagnosis and treatment of patients.Understanding Sensitivity
Definition and Importance
Sensitivity is a statistical measure that determines the proportion of true positive results in a population that has a specific condition or disease. It is a critical parameter in medical diagnosis, clinical trials, and other fields where accuracy is essential. Sensitivity measures the ability of a test to correctly identify individuals with a particular condition. It is calculated by dividing the number of true positives by the sum of true positives and false negatives.
Sensitivity is an essential metric in medical diagnosis because it helps to identify patients who may have a particular condition. A test with high sensitivity is useful when a false negative result could have serious consequences, such as in the case of a life-threatening disease like cancer. In such cases, a high sensitivity test is necessary to ensure that all patients with the disease are identified and treated promptly.
Sensitivity vs. Specificity
Sensitivity and specificity are two metrics used to evaluate the performance of diagnostic tests. While sensitivity measures the ability of a test to correctly identify individuals with a particular condition, specificity measures the ability of a test to correctly identify individuals without the condition.
A high sensitivity test is desirable when false negatives are unacceptable, while a high specificity test is desirable when false positives are unacceptable. For example, a pregnancy test should have high sensitivity to avoid false negatives, while a test for drug abuse should have high specificity to avoid false positives.
In conclusion, sensitivity is a crucial metric in medical diagnosis and other fields where accuracy is essential. It measures the ability of a test to correctly identify individuals with a particular condition and is necessary to ensure that all patients with the disease are identified and treated promptly. When evaluating diagnostic tests, sensitivity should be considered alongside specificity to determine the test's overall performance.The Basics of Calculation
Confusion Matrix Overview
Before calculating sensitivity, it's important to understand the concept of a confusion matrix. A confusion matrix is a table that is used to evaluate the performance of a classification model. It displays the number of true positives, true negatives, false positives, and false negatives.
The table is divided into two categories: predicted values and actual values. The predicted values are the values that the model predicts, while the actual values are the true values.
True Positives and False Negatives
To calculate sensitivity, you need to know the number of true positives and false negatives. True positives are the cases where the model predicted a positive result, and the actual result was also positive. False negatives are the cases where the model predicted a negative result, but the actual result was positive.
Once you have the number of true positives and false negatives, you can use the following formula to calculate sensitivity:
Sensitivity = True Positives / (True Positives + False Negatives)
By understanding the basics of a confusion matrix and the concept of true positives and false negatives, you can accurately calculate sensitivity.Step-by-Step Calculation
Gathering Data
Before calculating sensitivity, it is essential to gather the necessary data. This includes the number of true positive cases (TP), false negative cases (FN), true negative cases (TN), and false positive cases (FP). These data points can be obtained from medical records, clinical trials, or other relevant sources.
Applying the Formula
Once the data has been gathered, sensitivity can be calculated using the following formula:
Sensitivity = TP / (TP + FN)
To calculate specificity, the following formula can be used:
Specificity = TN / (TN + FP)
It is important to note that sensitivity and specificity are characteristics of the test and not the population. Therefore, the number of people in the population does not affect the calculation.
To illustrate the calculation of sensitivity, consider the following example:
Suppose a clinical trial is conducted to test the efficacy of a new drug. The trial involves 100 patients, of which 60 have the disease and 40 do not. The test correctly identifies 45 of the 60 patients with the disease and incorrectly identifies 10 of the 40 patients without the disease.
Using the formula above, the sensitivity of the test can be calculated as follows:
Sensitivity = 45 / (45 + 15) = 0.75
Therefore, the sensitivity of the test is 0.75 or 75%.
In conclusion, calculating sensitivity requires gathering the necessary data and applying the appropriate formula. By following these steps, healthcare professionals and researchers can accurately assess the effectiveness of diagnostic tests and treatments.Interpreting Results
Analyzing Sensitivity Outcomes
After calculating sensitivity, it is important to analyze the outcomes to determine the accuracy of the test. A high sensitivity result indicates that the test is effective at identifying individuals who have the condition being tested for. However, a high sensitivity result may also result in a high number of false positives, which can lead to unnecessary treatment and costs.
On the other hand, a low sensitivity result indicates that the test may miss individuals who have the condition being tested for. This can lead to delayed treatment and potentially negative health outcomes. Therefore, it is important to consider the sensitivity result in conjunction with other metrics, such as specificity and positive predictive value, to determine the overall accuracy of the test.
Comparing to Other Metrics
When interpreting sensitivity results, it is important to compare them to other metrics to determine the overall accuracy of the test. Specificity, for example, measures the proportion of true negatives among individuals without the condition being tested for. A high specificity result indicates that the test is effective at identifying individuals who do not have the condition being tested for.
Positive predictive value (PPV) measures the proportion of true positives among individuals who test positive for the condition being tested for. A high PPV result indicates that the test is effective at identifying individuals who have the condition being tested for.
Comparing sensitivity to other metrics can help provide a more complete picture of the accuracy of the test. It is important to consider all metrics when interpreting test results to ensure accurate diagnosis and treatment.Use Cases
Clinical Diagnostics
Sensitivity is a critical metric in clinical diagnostics. A test with high sensitivity is useful for ruling out a disease, while a test with low sensitivity is useful for ruling in a disease. For example, a pregnancy test with high sensitivity will detect pregnancy in almost all pregnant women, while a test with low sensitivity may miss some pregnancies.
Sensitivity is also important in cancer screening. A test with high sensitivity will detect most cancers, while a test with low sensitivity may miss some cancers, leading to false negatives. False negatives can be dangerous, as they can lead to delayed treatment and worse outcomes.
Data Science Applications
Sensitivity is also used in data science applications, particularly in machine learning and predictive modeling. In these applications, sensitivity is used to evaluate the performance of a model in detecting positive cases. For example, in fraud detection, a model with high sensitivity will detect most cases of fraud, while a model with low sensitivity may miss some cases.
Sensitivity is also used in medical research to evaluate the performance of diagnostic tests. For example, in a study evaluating a new diagnostic test for a disease, sensitivity is used to determine how well the test detects the disease in patients with the disease.
In summary, sensitivity is a critical metric in clinical diagnostics and data science applications. It is used to evaluate the performance of diagnostic tests and predictive models in detecting positive cases. A test or model with high sensitivity is useful for ruling out a disease or detecting positive cases, while a test or model with low sensitivity is useful for ruling in a disease or detecting negative cases.Improving Sensitivity
Adjusting Thresholds
One way to improve sensitivity is by adjusting the thresholds of the diagnostic test [1]. The threshold is the value that determines whether a test result is positive or negative. By lowering the threshold, more cases will be identified as positive, which can increase sensitivity. However, this may also increase the number of false positives, which can reduce specificity. Therefore, it is important to find a balance between sensitivity and specificity when adjusting thresholds.
Enhancing Data Quality
Another way to improve sensitivity is by enhancing the quality of the data used in the diagnostic test. This can be achieved by improving the accuracy and precision of the measurements, reducing measurement errors, and minimizing bias [2]. Collecting larger sample sizes can also improve the reliability of the results and reduce the likelihood of false negatives.
In addition, using multiple diagnostic tests can also increase sensitivity. This is because different tests may have different strengths and weaknesses, and combining them can improve overall accuracy [3]. However, this approach may also increase the cost and complexity of the diagnostic process.
Overall, improving sensitivity requires a careful balance between adjusting thresholds and enhancing data quality. By carefully considering these factors, healthcare professionals can improve the accuracy and reliability of diagnostic tests, and ultimately improve patient outcomes.
References:
Penn State University. (n.d.). 11.3 - Sensitivity, Specificity, Positive Predictive Value, and Negative Predictive Value. Retrieved June 29, 2024, from https://online.stat.psu.edu/stat507/lesson/11/11.3-0
Omni Calculator. (n.d.). Sensitivity and Specificity Calculator. Retrieved June 29, 2024, from https://www.omnicalculator.com/statistics/sensitivity-and-specificity
Statology. (n.d.). Positive Predictive Value vs. Sensitivity: What's the Difference? Retrieved June 29, 2024, from https://www.statology.org/positive-predictive-value-vs-sensitivity/
Frequently Asked Questions
What is the formula for calculating sensitivity in diagnostic tests?
Sensitivity is the proportion of true positive results among all individuals who have the condition being tested. The formula for sensitivity is True Positives / (True Positives + False Negatives) x 100%.
How do you determine sensitivity from true positive and false negative rates?
Sensitivity is calculated by dividing the number of true positive results by the lump sum loan payoff calculator (bbs.xiaoditech.com) of true positive and false negative results.
What methods are used to measure sensitivity in clinical research?
Sensitivity can be measured in clinical research through a variety of methods, including laboratory tests, imaging studies, and physical examinations. The method used will depend on the specific condition being tested for and the available resources.
How can sensitivity and specificity be calculated from a confusion matrix?
A confusion matrix is a table used to evaluate the performance of a binary classification model. Sensitivity can be calculated by dividing the number of true positive results by the sum of true positive and false negative results. Specificity can be calculated by dividing the number of true negative results by the sum of true negative and false positive results.
What is the process for computing sensitivity in relation to positive predictive value?
Sensitivity is the proportion of true positive results among all individuals who have the condition being tested. Positive predictive value is the proportion of true positive results among all individuals who test positive for the condition. To compute sensitivity in relation to positive predictive value, one must first calculate sensitivity and then divide the number of true positive results by the sum of true positive and false positive results.
How is sensitivity used alongside negative predictive value to assess test performance?
Sensitivity and negative predictive value are used together to assess the performance of a diagnostic test. Sensitivity measures the proportion of true positive results among all individuals who have the condition being tested, while negative predictive value measures the proportion of true negative results among all individuals who do not have the condition. Together, these measures can help determine the accuracy of a diagnostic test.
Местоположение
Род деятельности